일 | 월 | 화 | 수 | 목 | 금 | 토 |
---|---|---|---|---|---|---|
1 | 2 | 3 | 4 | 5 | ||
6 | 7 | 8 | 9 | 10 | 11 | 12 |
13 | 14 | 15 | 16 | 17 | 18 | 19 |
20 | 21 | 22 | 23 | 24 | 25 | 26 |
27 | 28 | 29 | 30 |
- pytorch
- 모두의 딥러닝 예제
- c언어
- 모두의 딥러닝
- 팀프로젝트
- 딥러닝 공부
- 딥러닝
- MFC 프로그래밍
- 해리스 코너 검출
- matlab 영상처리
- 케라스 정리
- 파이토치 강의 정리
- tensorflow 예제
- 미디언 필터링
- 파이토치 김성훈 교수님 강의 정리
- 영상처리
- pytorch zero to all
- 컴퓨터 비전
- 가우시안 필터링
- c++공부
- 파이토치
- c언어 정리
- TensorFlow
- 김성훈 교수님 PyTorch
- Pytorch Lecture
- C언어 공부
- 딥러닝 스터디
- 골빈해커
- object detection
- c++
- Today
- Total
목록전체 글 (235)
ComputerVision Jack
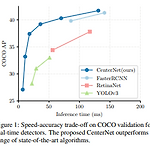
Objects at Points Abstract 대부분의 성능 좋은 object detectors는 가능성 있는 방대한 object location 리스트를 열거하고 각각을 분류한다. 이러한 방법은 낭비이며, 비효율적이고 추가적인 post-processing 필요로 한다. 논문에선 다른 접근 방법을 취한다. 객체를 single point 추론할 수 있게 모델을 설계한다. 해당 detector는 center point 찾기 위해 keypoint-estimation 진행하고, 서로 다른 모든 object properies에 대해 regression 진행한다. center point 기반의 접근 방법인 CenterNet은 end-to-end 구별이 가능하며 bounding box 기반인 detector 보다 ..
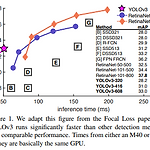
YOLOv3: An Incremental Improvement Abstract YOLO에 대해 더 좋은 성능을 할 수 있게 설계를 약간 변경하여 단단하게 만들었다. 또한 이 새로운 network 훈련 시켰다. 지난 YOLO 보다 약간 커졌지만 더 정확해졌다. YOLOv3 여전히 빠르다. 320 x 320 resolution에서 22 ms 나온다. SSD 만큼 정확하면서 3배 빠르다. YOLO: Real-Time Object Detection YOLO: Real-Time Object Detection YOLO: Real-Time Object Detection You only look once (YOLO) is a state-of-the-art, real-time object detection system...
Exception in thread Thread -3: 해당 오류는 Classification Task 진행하던 도중에 만나게 되었다. pytorch lightning module 사용하여 Trainer 정의할 때, worker 설정과 관련한 오류로, worker 맞게 설정하거나 낮추면 해결 가능하다. trainer = pl.Trainer( max_epochs=cfg['epochs'], logger=TensorBoardLogger(cfg['save_dir'], make_model_name(cfg)), gpus=cfg['gpus'], accelerator='ddp' if platform.system() != 'Windows' else None, plugins=DDPPlugin() if platform.sy..
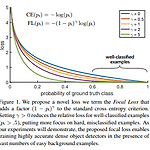
Focal Loss for Dense Object Detection Abstract 객체의 위치에 대한 가능성에 대해 regular, dense sampling 적용하는 one-stage detectors는 빠르고 간단하다. 하지만 two-stage detectors의 정확도 측면에는 다가가지 못했다. 앞의 이유 중 가장 큰 원인은 dense detectors 학습 시킬 때, foreground와 background 간의 class imbalance것을 발견했다. 이러한 원인을 해결하기 위해 cross entropy loss 수정하여 잘 분류된 예제에 대해선 down-weights 적용한다. 새로운 Focal Loss는 hard examples 집합에 초점을 두어 방대한 negative 집합이 학습 기간..
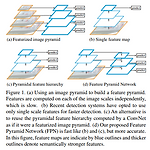
Feature Pyramid Networks for Object Detection Abstract feature pyramids는 다른 크기의 object detection 위한 recognition systems에서 기본적인 요소이다. 그러나 최근 deep learning 사용하는 object detection은 feature pyramids 사용을 피해왔다. 왜냐하면 compute와 memory가 방대해지기 때문이다. 논문에서 저자들은 deep convolutional networks에 포함되어 있는 multi-scale pyramidal hierarchy 활용하여 비용 측면을 고려한 feature pyramids 설계한다. top-down 구조는 문자 그대로 모든 크기에서 높은 단계의 semanti..
TypeError: Conv2d() received an incalid combination of arguments 해당 오류는 Classification Task 진행하던 도중에 만나게 되었다. Module에 관련된 오류로 Conv2d 자체의 문제 보단 input으로 들어가는 데이터와 관련된 오류이다. # 이미지 가시화 진행 return {'img': transformed, 'class': label} # 학습 적용시 return transformed, label 따라서 visualize 진행할 경우 dict 자료 구조로 반환을 넘겨주면 되지만 학습시엔 label 자체를 넘겨줘야한다.
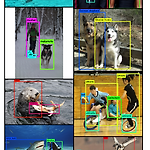
YOLO 90000: Better, Faster, Stronger Abstract real-time object detection system YOLO9000 소개한다. 이는 9000개의 object categories에 대해 검출 할 수 있다. 첫 번째로 이전 방법의 새로움과 결점을 토대로 YOLO detection 방법의 다양한 향상을 제안한다. 새로운 방법을 사용하면서, multi-scale training method 똑같이 YOLOv2 모델에 적용하면서 다양한 크기와 speed와 accuracy 사이의 tradeoff에 대해 쉽게 동작할 수 있다. 마지막으로 object detection과 classification 사이의 jointly train method 제안한다. Introduction ..
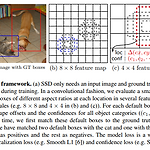
SSD: Single Shot MultiBox Detector Abstract single deep neural network 사용하여 image에서 object detection 진행하는 방법을 제시한다. SSD 해당 접근 방법은 bounding box의 output space에 대해 feature map 위치에서 다른 ratios와 scales 갖는 default box 연산한다. prediction time에 network는 각 default box안의 object category에 대한 scores 발생 시키고, object의 shape에 잘 부합하는 box 생산한다. 추가적으로 network는 multiple feature maps에서 prediction 값을 결합한다. 이는 다양한 object..