일 | 월 | 화 | 수 | 목 | 금 | 토 |
---|---|---|---|---|---|---|
1 | 2 | 3 | 4 | |||
5 | 6 | 7 | 8 | 9 | 10 | 11 |
12 | 13 | 14 | 15 | 16 | 17 | 18 |
19 | 20 | 21 | 22 | 23 | 24 | 25 |
26 | 27 | 28 | 29 | 30 | 31 |
Tags
- 케라스 정리
- 골빈해커
- 모두의 딥러닝
- TensorFlow
- tensorflow 예제
- 딥러닝 스터디
- 딥러닝 공부
- Pytorch Lecture
- 팀프로젝트
- 해리스 코너 검출
- 딥러닝
- c++공부
- 파이토치 강의 정리
- 미디언 필터링
- c++
- c언어 정리
- 가우시안 필터링
- 컴퓨터 비전
- c언어
- 모두의 딥러닝 예제
- object detection
- 파이토치
- 영상처리
- pytorch
- MFC 프로그래밍
- pytorch zero to all
- 파이토치 김성훈 교수님 강의 정리
- C언어 공부
- 김성훈 교수님 PyTorch
- matlab 영상처리
Archives
- Today
- Total
목록Classification backbone (1)
ComputerVision Jack
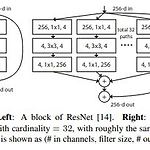
Aggregated Residual Transformations for Deep Neural Networks Abstract 논문에서 소개하는 Network는 같은 Topology에 대해 변형 시킨 Building Block 반복하여 설계하였다. 이 설계는 적은 Hyper-Parameter 통해서 동종의 Multi-Branch한 구조의 결과로 나타났다. 앞에서 소개한 전략은 "Cardinality" (the size of the set of transformations) 새로운 Dimension 생성한다. 이 Dimension은 Depth와 Width Dimension 외에도 필수적인 요소이다. 게다가 Capacity 위해 Network 깊고, 넓게 만드는 것 보다, Cardinality 증가 시키는 것..
Reading Paper/Classification Networks
2021. 12. 21. 09:55